Need of Explainable AI – Explainable AI
Artificial intelligence has the ability to automate judgments, and the outcomes of such decisions may have both beneficial and bad effects on businesses. It is essential to have an understanding of how AI comes to its conclusions, just as it is essential to have this understanding when recruiting decision is made for the business. A great number of companies are interested in using AI, but are hesitant to hand over decision-making authority to the model or AI simply because they do not yet trust the model. Explainability is beneficial in this regard since it offers insights into the decision-making process that models use. Explainable AI is a crucial component in the process of applying ethics to the usage of AI in business. Explainable AI is predicated on the notion that AI-based applications and technology should not be opaque “black box” models that are incomprehensible to regular people. Figure 10.1 shows the difference between AI and Explainable AI:
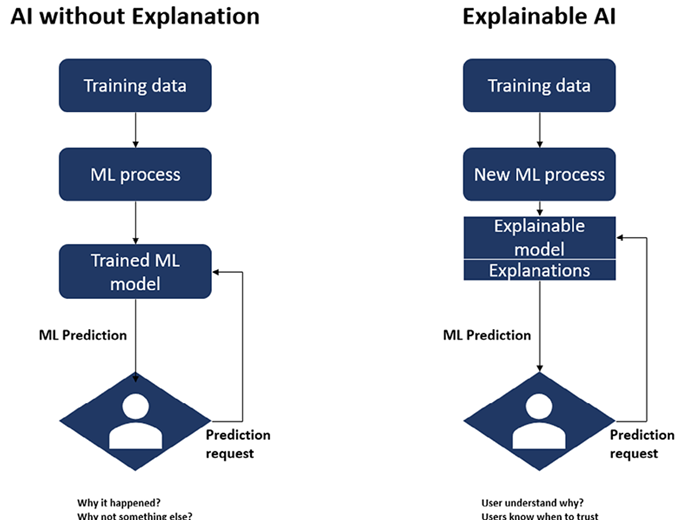
In the majority of the scenarios developing complex models is far easier than convincing stakeholders that the model is capable of producing decisions that are superior to those produced by humans. It is not the same thing as having greater accuracy scores or a lower RMSE to make a better judgment. A correct conclusion may be reached by providing accurate data as input. In many cases, the person making the choice is the one who needs to comprehend it. For them to feel at ease handing over the decision-making to the model, they need to understand how the model came to its conclusions.
Explainable AI is essential to the development of responsible AI because it offers an adequate amount of transparency and responsibility for the choices made by complicated AI systems. This is of utmost importance when it comes to artificial intelligence systems that have a substantial influence on the lives of people.
Explainable AI of Vertex AI provides explanations that are either feature-based or example-based in order to give a better understanding of how models make decisions. Anyone who builds or uses machine learning will gain new abilities if they learn how a model behaves and how it is influenced by its training dataset. These new abilities will allow users to improve their models, increase their confidence in their predictions, and understand when and why things work.